Commit 024cd82
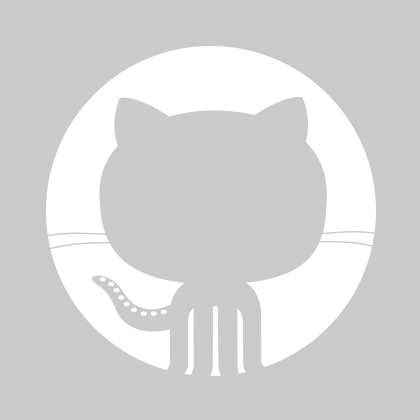
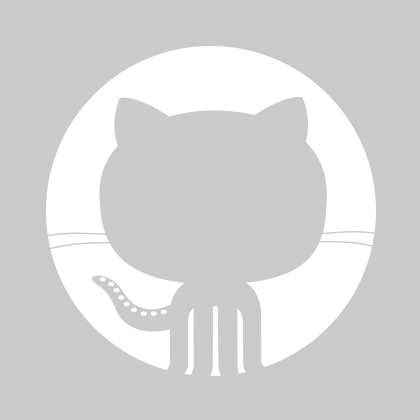
Milvus-doc-bot
Milvus-doc-bot
1 parent 7175b07 commit 024cd82
File tree
5 files changed
+24
-10
lines changed- v0.10.2
- assets
- site
- en/reference
- zh-CN/reference
5 files changed
+24
-10
lines changed9.4 KB
Loading
Loading
Loading
Original file line number | Diff line number | Diff line change | |
---|---|---|---|
| |||
27 | 27 |
| |
28 | 28 |
| |
29 | 29 |
| |
30 |
| - | |
31 |
| - | |
32 |
| - | |
| 30 | + | |
33 | 31 |
| |
34 | 32 |
| |
35 | 33 |
| |
36 |
| - | |
| 34 | + | |
37 | 35 |
| |
38 | 36 |
| |
39 | 37 |
| |
40 | 38 |
| |
41 |
| - | |
| 39 | + | |
42 | 40 |
| |
43 | 41 |
| |
| 42 | + | |
| 43 | + | |
| 44 | + | |
| 45 | + | |
| 46 | + | |
| 47 | + | |
| 48 | + | |
| 49 | + | |
| 50 | + | |
44 | 51 |
| |
45 | 52 |
| |
46 | 53 |
| |
|
Original file line number | Diff line number | Diff line change | |
---|---|---|---|
| |||
27 | 27 |
| |
28 | 28 |
| |
29 | 29 |
| |
30 |
| - | |
| 30 | + | |
31 | 31 |
| |
32 |
| - | |
| 32 | + | |
33 | 33 |
| |
34 |
| - | |
35 | 34 |
| |
36 |
| - | |
| 35 | + | |
37 | 36 |
| |
38 | 37 |
| |
39 | 38 |
| |
40 | 39 |
| |
41 |
| - | |
| 40 | + | |
42 | 41 |
| |
43 | 42 |
| |
| 43 | + | |
| 44 | + | |
| 45 | + | |
| 46 | + | |
| 47 | + | |
| 48 | + | |
| 49 | + | |
| 50 | + | |
44 | 51 |
| |
45 | 52 |
| |
46 | 53 |
| |
|
0 commit comments